Friday, September 4, 2020
Exploiting symmetry in structured data is a powerful way to improve learning and generalization ability of AI systems, and extract more information, in applications from vision and NLP to robotics. This is exemplified by convolutional neural nets, which are an ubiquitous architecture. Recently, there has been a great deal of progress to develop improved equivariant and invariant learning architectures, as well as improved data augmentation methods. There has also been progress on the theoretical foundations of the area, from the perspectives of statistics and optimization. The notion of adding data via data augmentation also arises in problems such as adversarial robustness. This workshop will bring together leading researchers in the area to discuss the state of the art of the field. The activity is part of the Center for Foundations of Information Processing at Penn, supported by NSF TRIPODS.
Due to Covid-19, the workshop will be held online as a Zoom webinar. While this means less in-person interaction, it also means that the talks are accessible to everyone for free, without the costs of travel.
Recorded talks are available as a Youtube playlist. Individual videos are also linked below. The link below takes you to the playlist in the “play all” mode.
Schedule
9:00am- Haggai Maron, Leveraging permutation group symmetries for designing equivariant neural networks
9:30am- Taco Cohen, Equivariant Networks and Natural Graph Networks
10:00am- Danilo J. Rezende, Generative Models and Symmetries
10:30am- Kilian Q. Weinberger, Learning with Marginalized Augmenation
11:00am- Mark van der Wilk, Learning Invariances through Backprop with Bayesian Model Selection
11:20am- Break
11:40am- Alexander Robey, Model-based Robust Deep Learning
12:00pm- Alejandro Ribeiro, Algebraic Neural Networks: Symmetry and Stability
12:20pm- Pratik Chaudhari, Learning with few labeled data
12:40pm- Carlos Esteves, Spin-Weighted Spherical CNNs
12:55pm- Jane H. Lee, A group-theoretic framework for data augmentation
1:10pm- Panel
1:55pm- Lunch Break
2:30pm- Fabio Anselmi, Neurally plausible mechanisms for learning selective and invariant representations
3:00pm- Christine Allen-Blanchette, LagNetViP: A Lagrangian Neural Network for Video Prediction
3:30pm- Tess E. Smidt, Unintended features of Euclidean symmetry equivariant neural networks
4:00pm- Greg Valiant, Amplifying Datasets: A Theoretical Perspective
4:30pm- Chelsea Finn, Meta-Learning Symmetries
5:00pm- Hongyang R. Zhang, Generalization Effects of Linear Transformations in Data Augmentation
Speakers
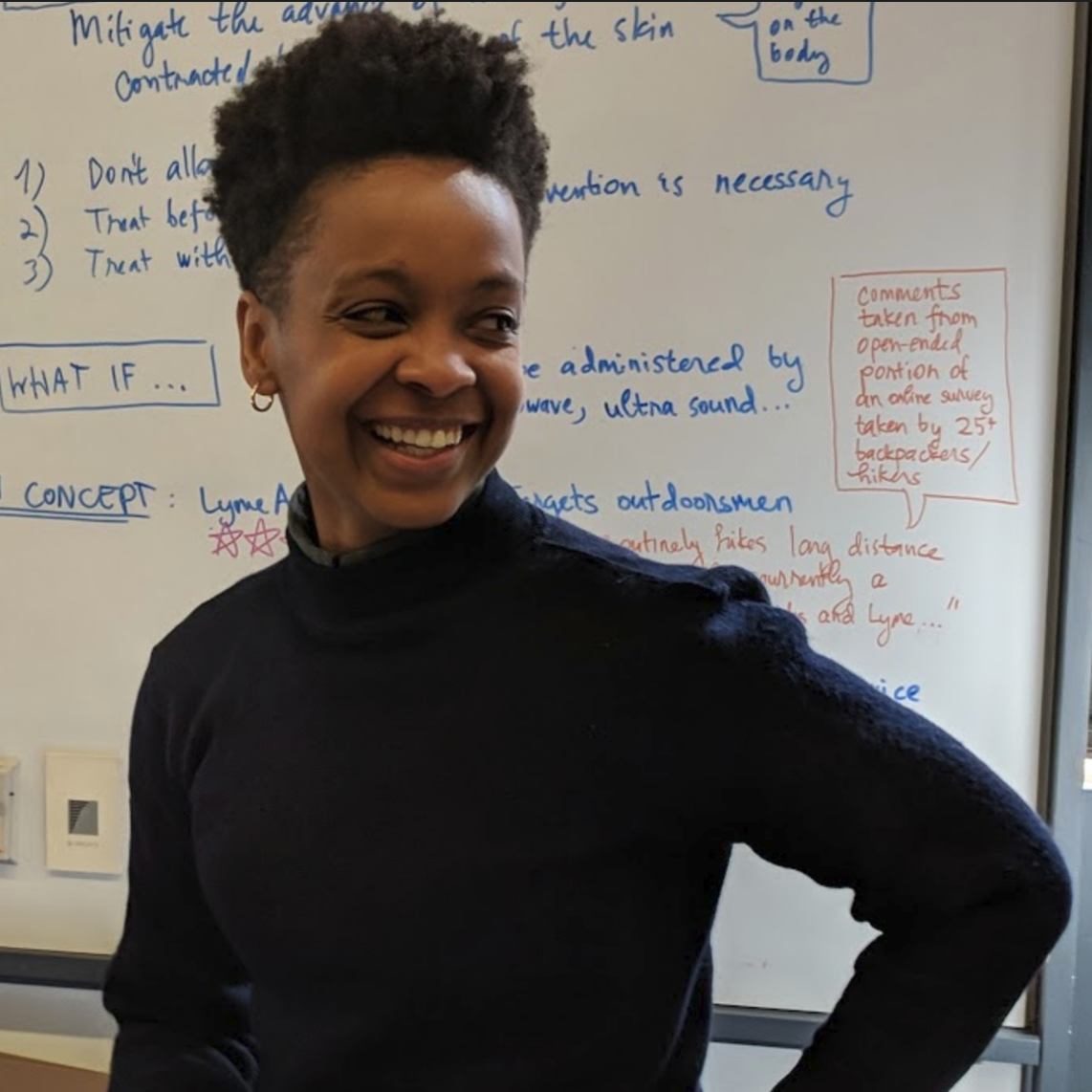
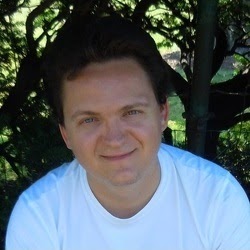
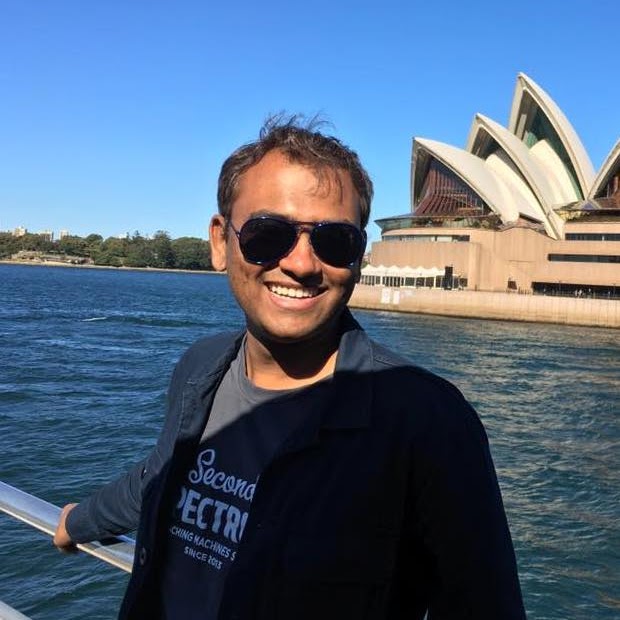
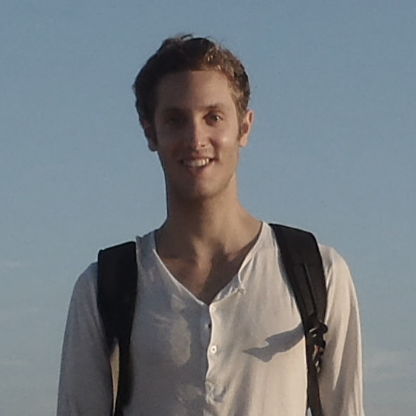
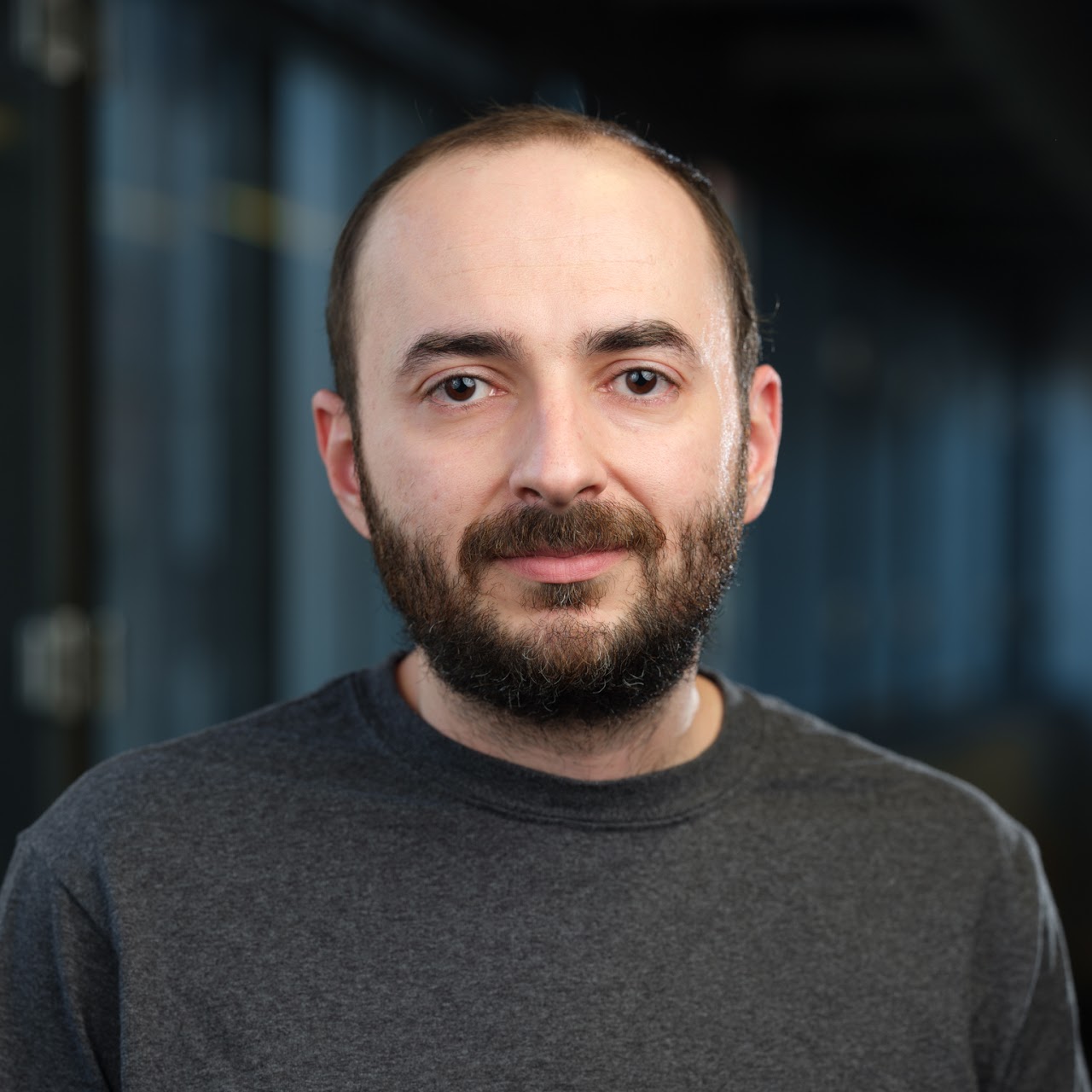
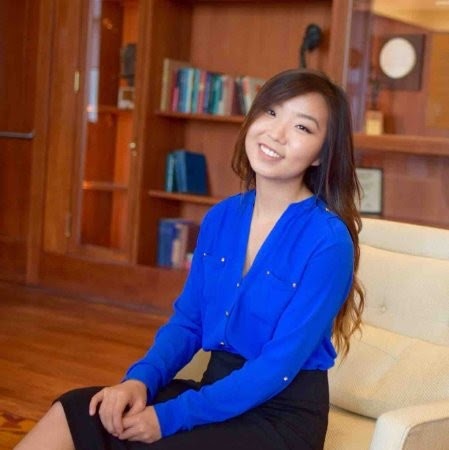
Jane H. Lee
Twitter/Yale University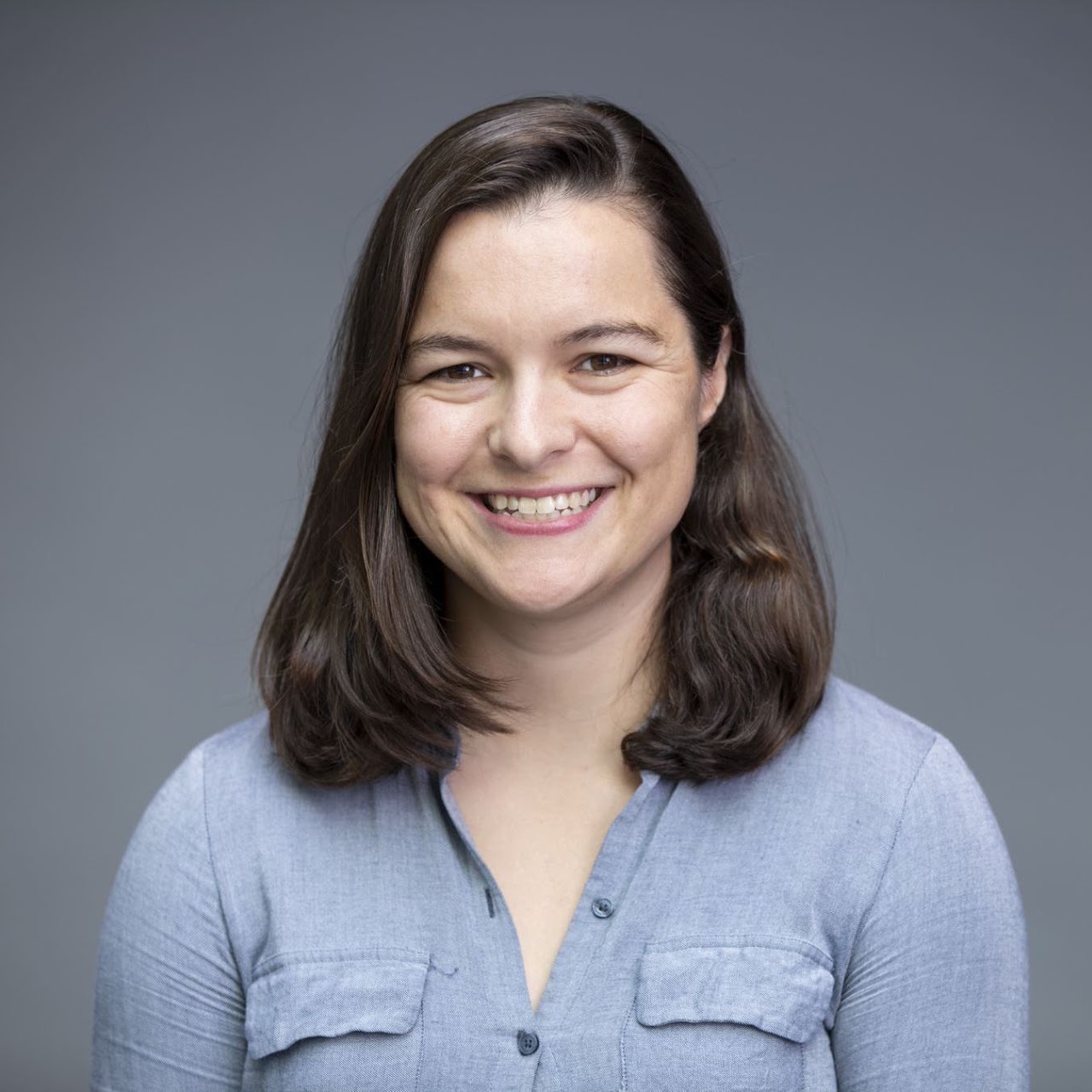
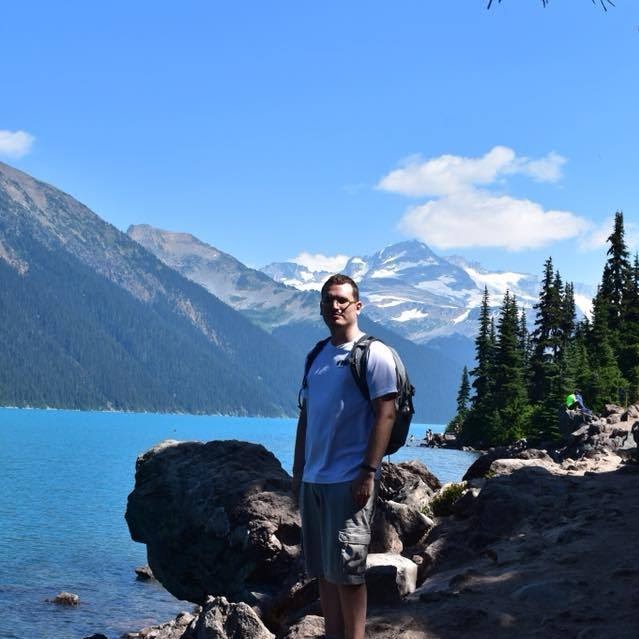
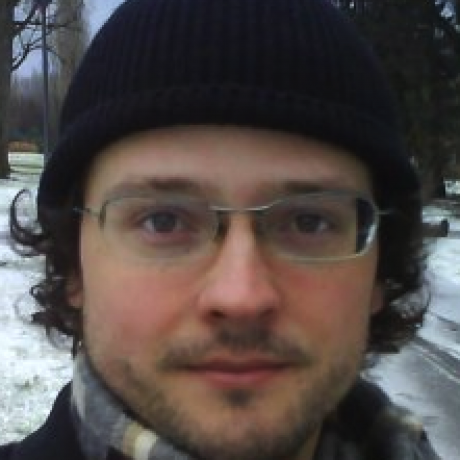
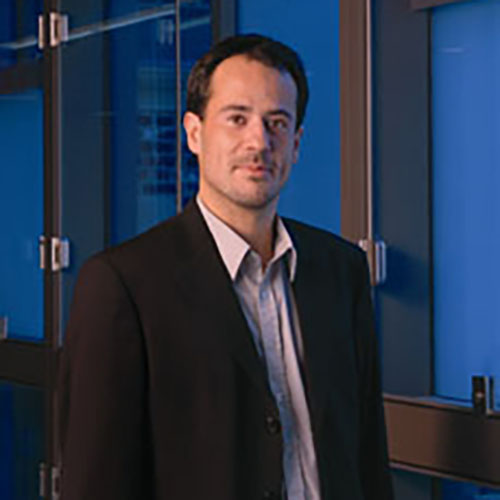
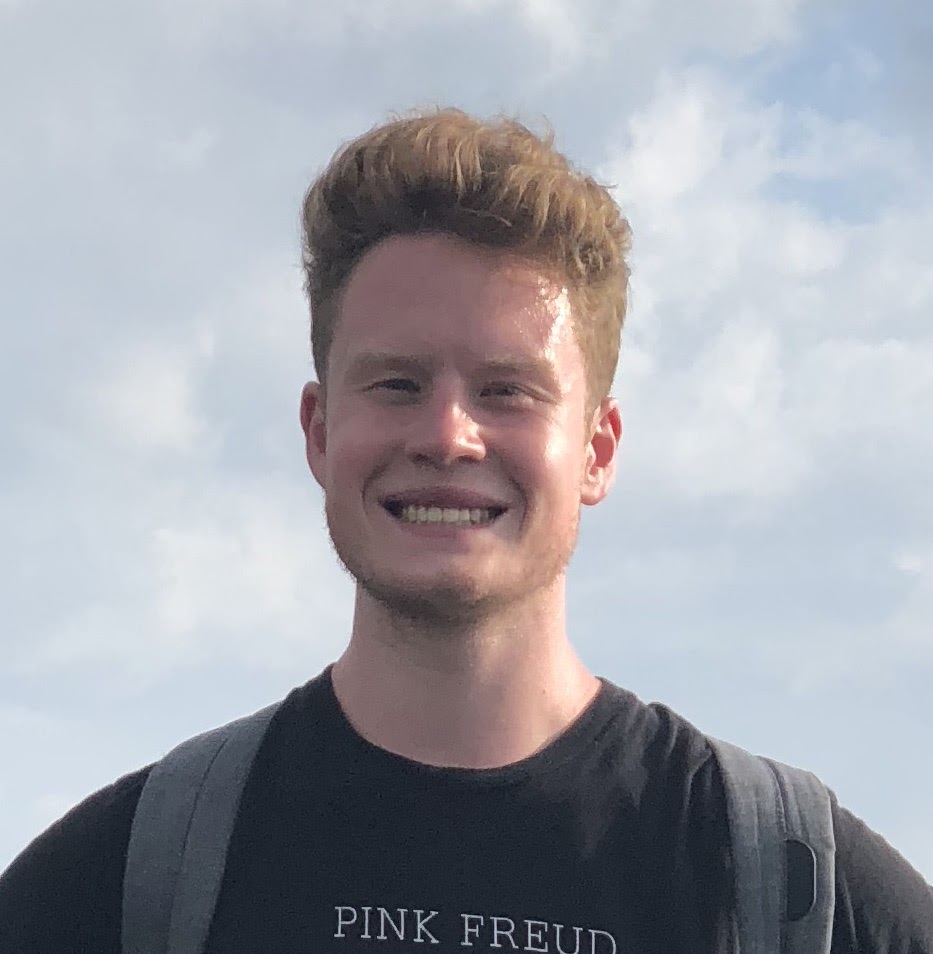
Alexander Robey
University of Pennsylvaniahttps://scholar.google.com/citations?user=V5NWZc8AAAAJ&hl=en
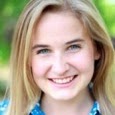
Tess E. Smidt
Lawrence Berkeley National Laboratoryhttps://crd.lbl.gov/departments/computational-science/ccmc/staff/alvarez-fellows/tess-smidt/
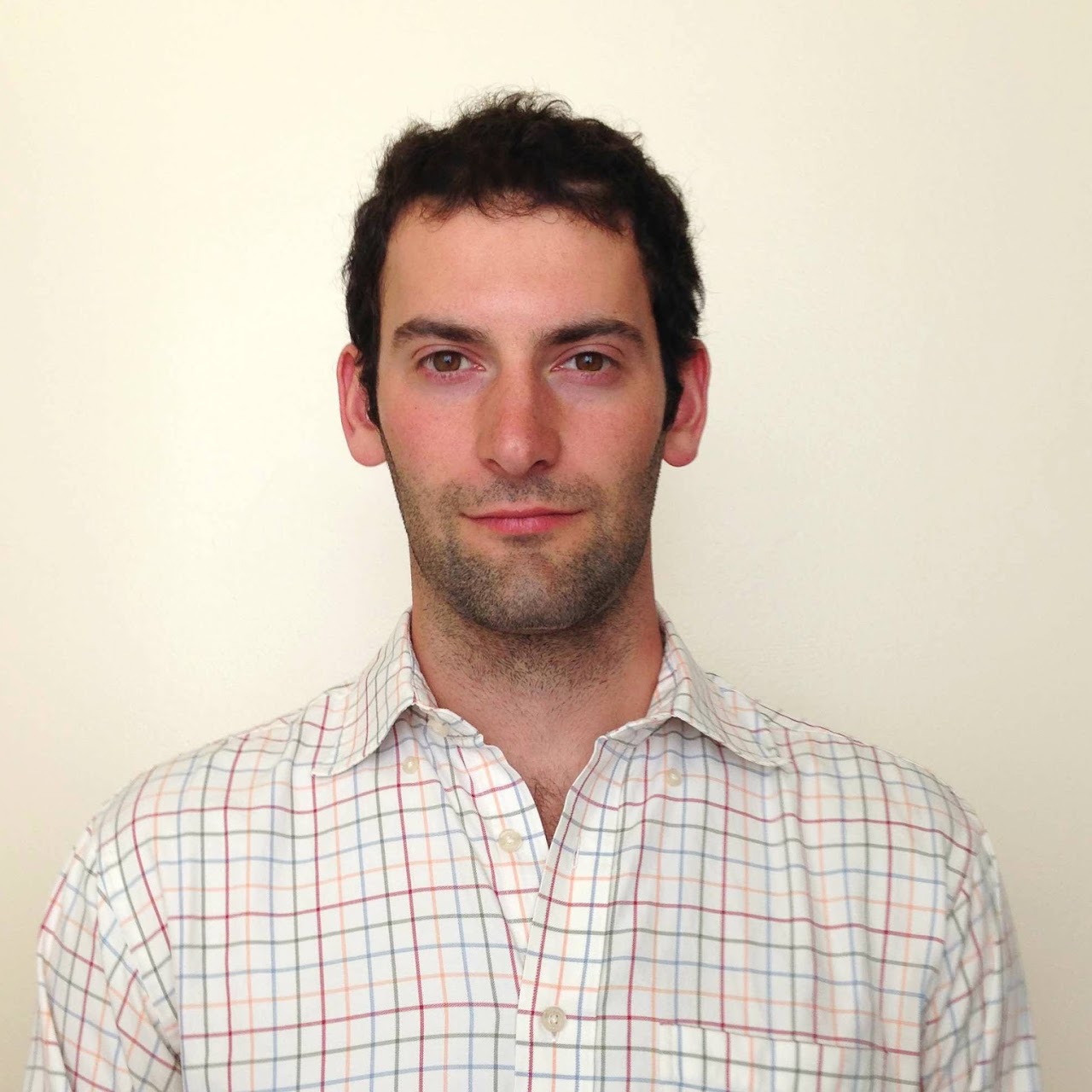
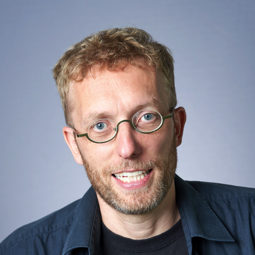
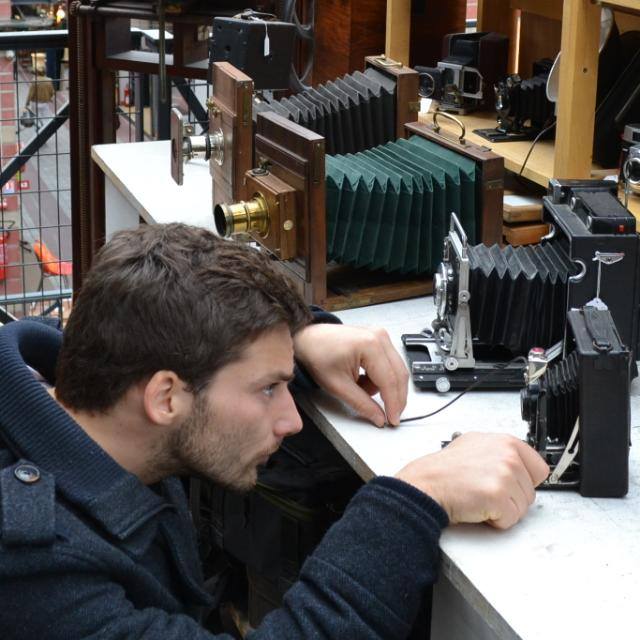
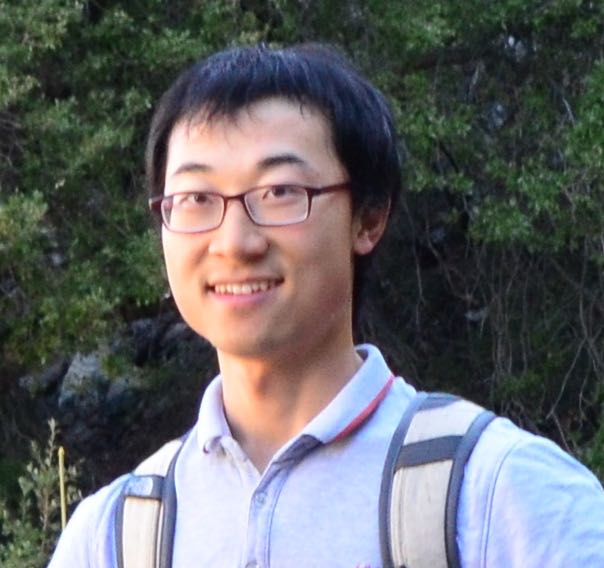
Contacts
Please contact Edgar Dobriban or Kostas Daniilidis with any questions.
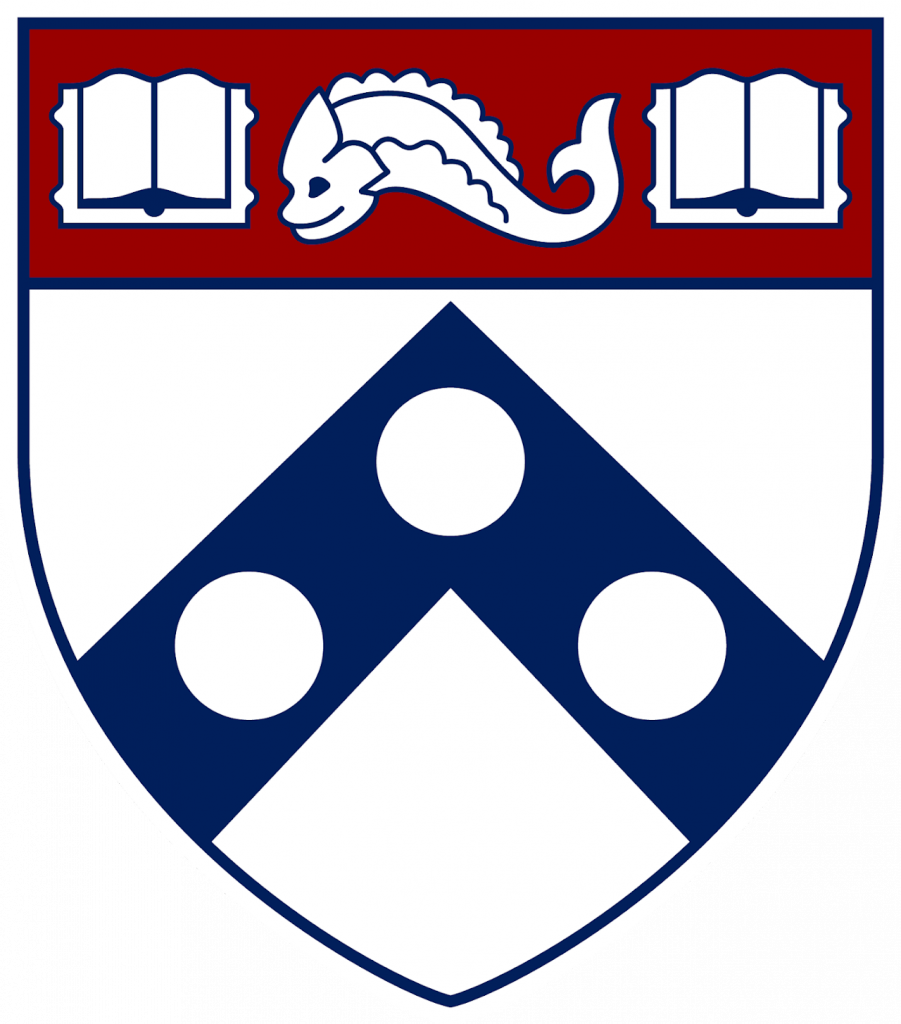
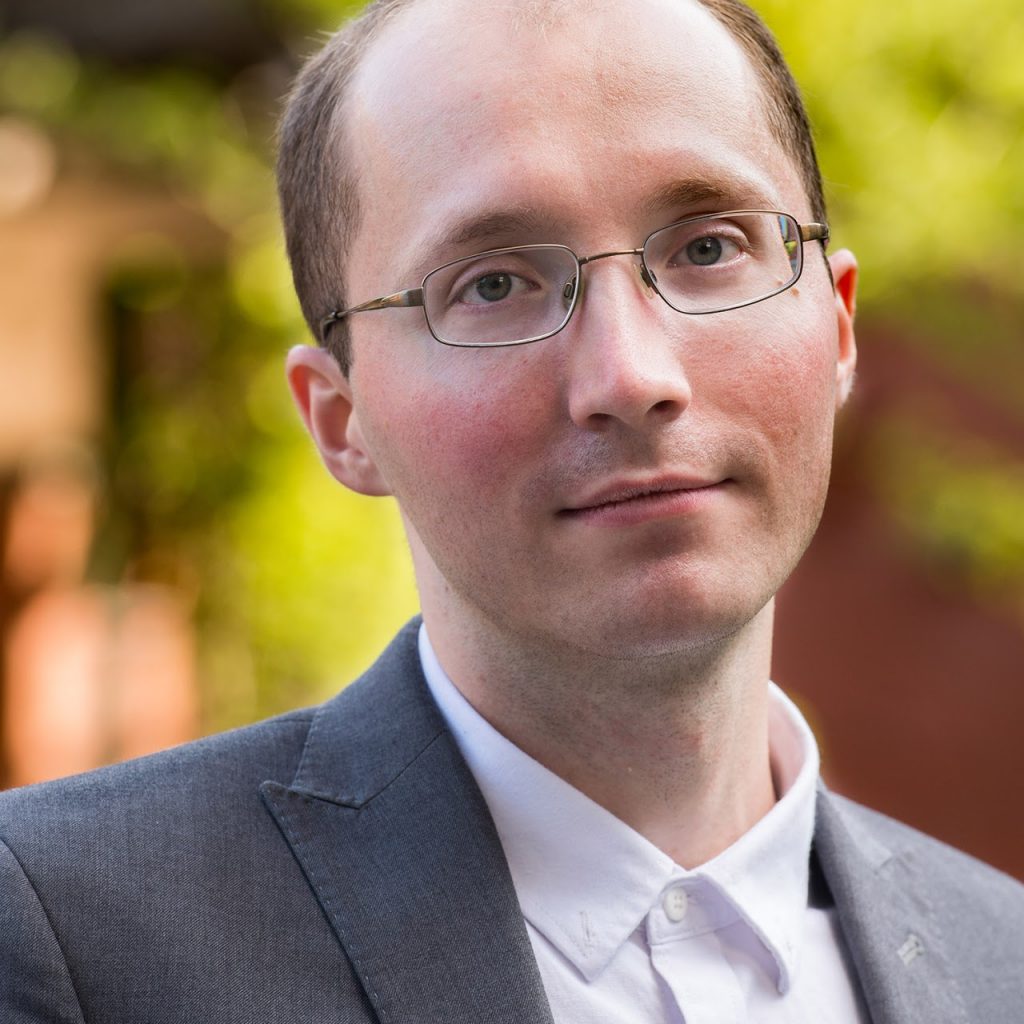
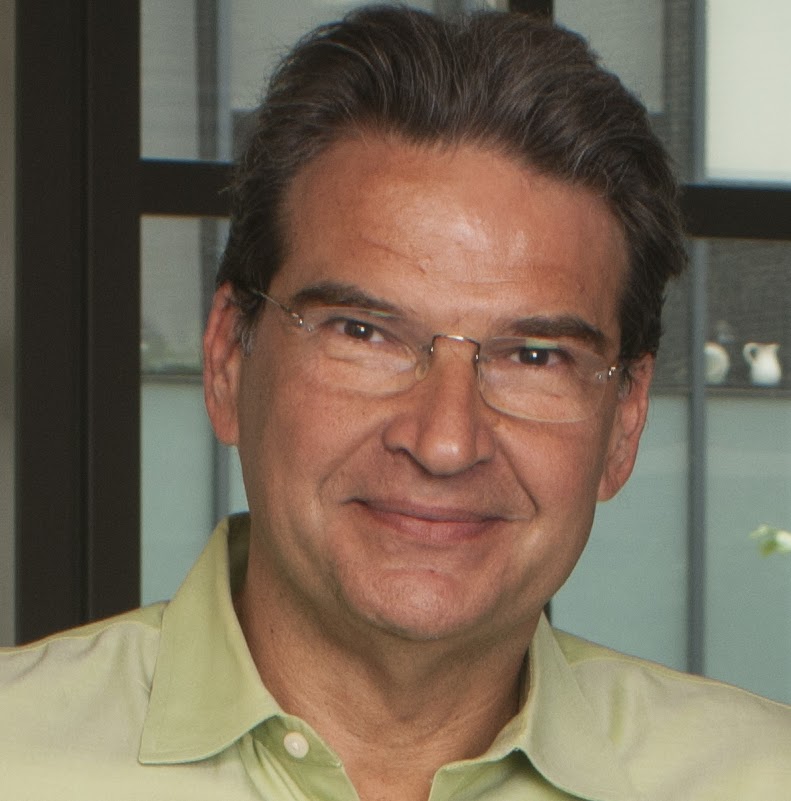
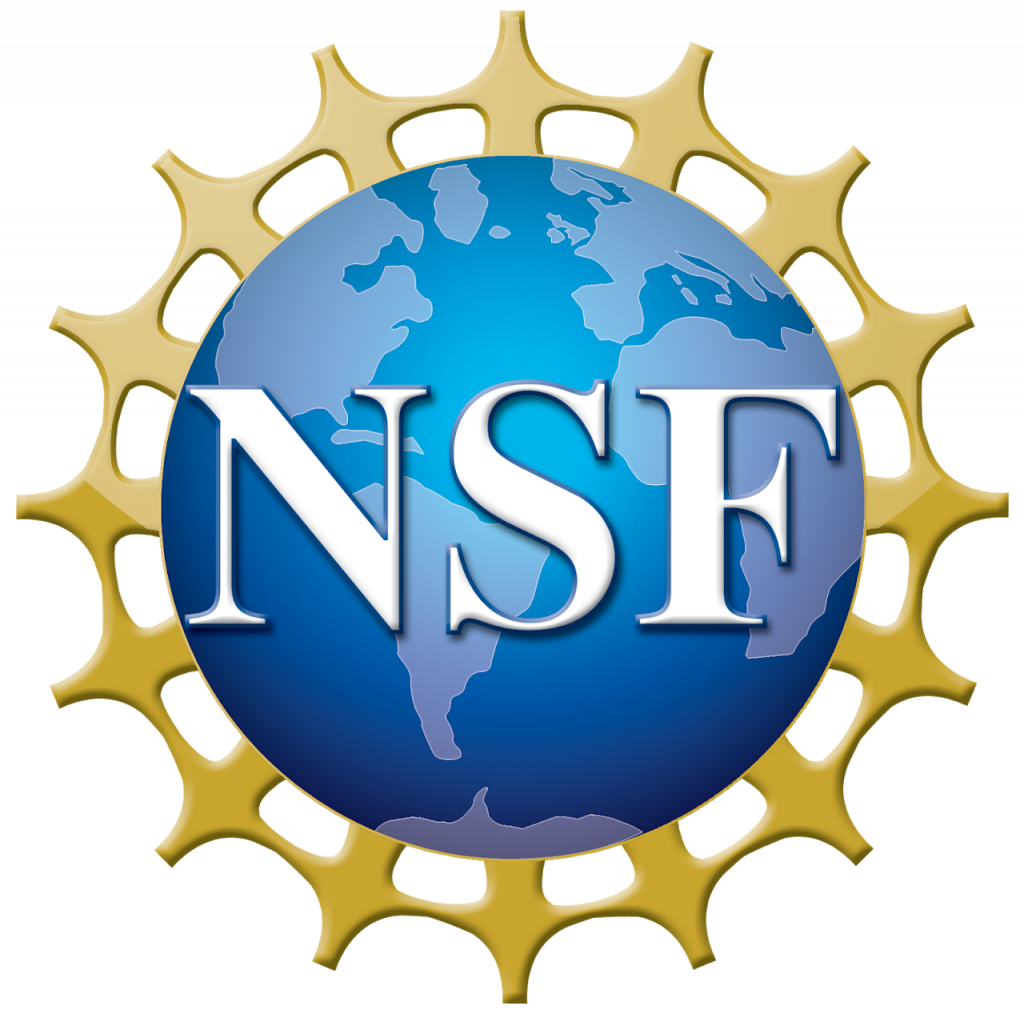